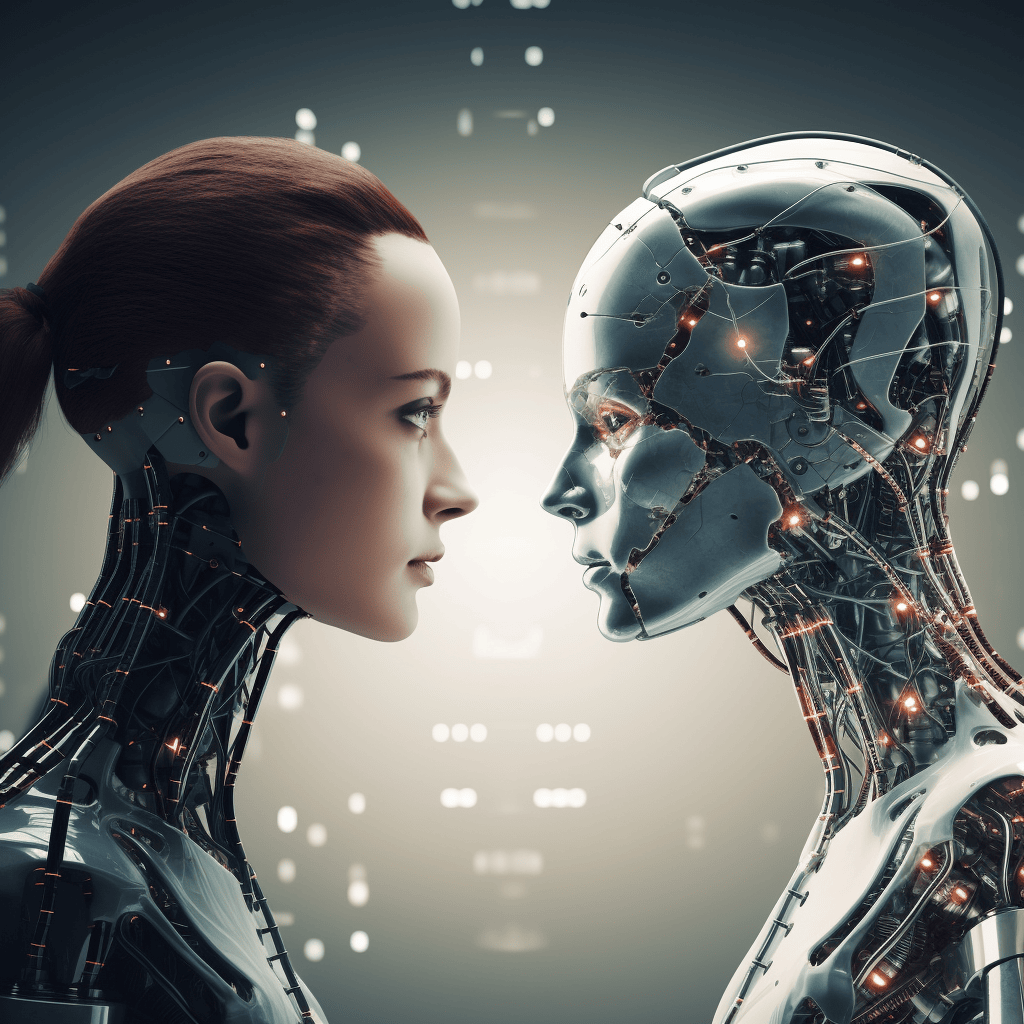
In today’s rapidly evolving technological landscape, understanding AI bias has become increasingly crucial. This comprehensive article dives deep into the causes and solutions surrounding AI bias, shedding light on the potential implications of biased algorithms. Join us on this enlightening journey as we unravel the intricacies of AI bias and explore practical approaches to mitigate its effects.
What is AI Bias?
AI Bias: Uncovering the Challenges of Biased Algorithms
AI bias refers to the systematic favoritism or discrimination exhibited by AI algorithms, resulting in unfair or unequal treatment of certain individuals or groups. This section delves into the intricacies of AI bias, exploring its causes, implications, and the need for effective solutions.
The Impact of AI Bias
Implications of AI Bias: Shaping Our Technological Landscape
The impact of AI bias can be profound, with far-reaching consequences that affect individuals, communities, and society as a whole. This section highlights the potential pitfalls of biased algorithms, emphasizing the need to address AI bias for the sake of fairness and equitable outcomes.
Understanding AI Bias: Causes and Solutions
Exploring the Causes of AI Bias: Data, Algorithms, and Human Factors
To effectively address AI bias, it is vital to comprehend its underlying causes. This section focuses on three primary sources of AI bias: data bias, algorithmic bias, and human bias. By understanding these causes, we can develop practical solutions to mitigate bias in AI systems.
Data Bias: The Role of Biased Training Data
Data bias, a significant contributor to AI bias, arises when training data contains inherent biases or reflects societal prejudices. This subsection explores the impact of data bias and highlights the importance of diverse and representative data collection to reduce bias in AI algorithms.
Algorithmic Bias: Unravelling the Decision-Making Process
Algorithmic bias occurs when the design or structure of an algorithm leads to biased outcomes. In this subsection, we delve into the intricacies of algorithmic bias, emphasizing the need for transparent and explainable algorithms that can be scrutinized and rectified to ensure fairness.
Human Bias: The Human Element in AI Development
Human bias, consciously or unconsciously embedded in AI systems by developers, is a significant concern. This subsection sheds light on the influence of human bias in AI development and the importance of diverse and inclusive teams to minimize bias.
The Consequences of AI Bias
Consequences of AI Bias: Unveiling the Ramifications
AI bias can have wide-ranging consequences, perpetuating discrimination, reinforcing stereotypes, and undermining trust. This section explores the potential negative impacts of AI bias, reinforcing the urgency to address this issue and create a more equitable technological landscape.
Addressing AI Bias: Practical Solutions
Mitigating AI Bias: Approaches for Fair and Ethical AI
To tackle AI bias effectively, practical solutions need to be implemented. This section highlights essential approaches that can help address AI bias and promote fairness and accountability in AI systems.
Diverse and Representative Data Collection: Towards Inclusive Algorithms
Collecting diverse and representative data is a crucial step in reducing AI bias. This subsection emphasizes the significance of inclusive data collection practices, enabling algorithms to better account for the needs and perspectives of diverse populations.
Transparent and Explainable Algorithms: Promoting Trust and Accountability
Developing transparent and explainable algorithms is vital to gain insights into decision-making processes. By promoting algorithmic transparency and explainability, stakeholders can assess the fairness of AI systems and take necessary actions to mitigate bias.
Continuous Monitoring and Evaluation: Ensuring Ongoing Fairness
Regular monitoring and evaluation are essential to identify and rectify bias in AI systems. This subsection emphasizes the importance of continuous oversight and assessment to proactively address bias and ensure ongoing fairness and accountability.
Ethical Frameworks and Guidelines: Guiding Responsible AI Development
Adhering to ethical frameworks and guidelines is crucial in promoting unbiased AI development. This subsection highlights the need for established ethical principles and best practices to guide developers in creating AI systems that prioritize fairness and societal well-being.
FAQs on Understanding AI Bias: Causes and Solutions
What is AI bias?
AI bias refers to the systematic favoritism or discrimination exhibited by AI algorithms, resulting in unfair treatment or unequal outcomes for certain individuals or groups.
How does AI bias occur?
AI bias can occur due to data bias, algorithmic bias, or human bias. Biased training data, flawed algorithms, and subjective decision-making during AI development can contribute to bias in AI systems.
Can AI algorithms be completely unbiased?
Achieving completely unbiased AI algorithms is a challenging task. While eliminating all forms of bias may be difficult, developers can strive to minimize bias and ensure fairness through appropriate measures and ethical practices.
Who is responsible for addressing AI bias?
Addressing AI bias is a collective responsibility that involves developers, data scientists, policymakers, and other stakeholders. It requires collaboration and proactive efforts from all parties involved in AI development and deployment.
How can diverse datasets help reduce AI bias?
Diverse datasets play a crucial role in reducing AI bias. By incorporating data from various demographic groups and ensuring representation, developers can train AI algorithms to be more inclusive and equitable.
Are there any legal frameworks to regulate AI bias?
Various countries and regions are recognizing the need for legal frameworks to regulate AI bias. These frameworks aim to ensure transparency, accountability, and fairness in AI systems, protecting individuals from discriminatory practices.
Conclusion
In conclusion, understanding AI bias is pivotal for the development of fair and unbiased AI systems. By comprehending its causes and implementing practical solutions, we can work towards mitigating the adverse effects of bias. Through diverse data collection, transparent algorithms, continuous monitoring, and adherence to ethical frameworks, we can foster a technological landscape that promotes equity and inclusivity.
As AI continues to shape our society, addressing AI bias becomes an increasingly critical endeavor. By raising awareness, collaborating, and adopting responsible practices, we can pave the way for a future where AI technologies empower all individuals and contribute to a more just and equitable world.